Vensim Ple Serial
Shareware Junction periodically updates pricing and software information of Vensim PLE v.5.11.2.1 full version from the publisher using pad file and submit from users. Software piracy is theft, Using crack, password, serial numbers, registration codes, key generators, cd key, hacks is illegal and prevent future development of Vensim PLE v.5.11.2.1 Edition. Download Vensim PLE 6.3 from our website for free. '.mdl' and '.vmf' are the extensions this PC software can open. Our antivirus analysis shows that this download is clean. The latest version of Vensim PLE can be downloaded for PCs running Windows XP/Vista/7/8/10, 32-bit. This tool was originally developed by Ventana Systems, Inc.
In order to make a sensible prediction on the air traffic flow management with conditions of wave-off and bolter, a system dynamic model for the night recovery operations of embarked aircrafts is built to ensure the adaption of air traffic flow with the capacity of air control at each phase of the recovery operations. The model aims at the characteristics of multiple feedbacks, delays and complex time varying, builds a stock flow diagram and operation model with impact factors of the night recovery system, and is simulated in Vensim(r) Personal Learning Edition 5.9. The simulation shows a reasonable prediction result for the night recovery of embarked aircrafts with conditions of bolter and wave-off and can provide a theoretical basis for scheduling the air traffic flow management of embarked aircrafts formation recovery. KEYWORDS: Air traffic flow management; Recovery; Embarked aircrafts formation; System dynamics; Complex system modeling. INTRODUCTION Aircraft Carrier Attack (CVA), with the ability of fast oversea power projection, becomes the main combat force of modern navies. For a large number of aircrafts in the process of formation recovery, secure, efficient and sequential air traffic flow managements are necessary to make full use of the capacity of airspace and flight deck, improve the utilization of time and space, and provide the latest information for the carrier-aircrafts system.
The issue on civil air traffic flow management has been well studied by researchers both at home and abroad. Taking the complexity of the air traffic management system ( ) into consideration, researchers presented 3 approaches to solve the air traffic flow management problem: air traffic capacity management ( ), ground holding management ( ) and flight schedule optimization ( ). Studied the problem of air traffic flow management in uncertain severe convective weather and established a dynamic reroute planning model based on genetic algorithms. In, an auction-based market method was proposed to solve the congestion problem of airspace in the stage of pre-tactic and tactic air traffic flow management, and a decision-making model for airline company was analyzed based on game theory. Used an integer optimization method to research the air traffic flow management while studied the preliminary benefits of the analysis of air traffic flow management. Studied a decision-support system of the tactical air traffic flow management. A dynamic model for air traffic flow management and a research about the flight deck scheduling based on distributed network were presented in and, respectively.
Designed an interactively local and global decision-support system for aircraft carrier deck scheduling. In, the stock flowchart and the mathematical model of the complex time-varying system for dynamic handling of embarked aircrafts were built based on system dynamics (SD) after discussion of the process of aircraft turnaround and transferring of embarked aircrafts among the flight deck, hangar and elevators. In, to make a reasonable schedule of recovery considering wave-off and bolter of aircrafts, an operation model on embarked aircraft recovery in good weather was built.
Presented a practical stochastic optimization model for air traffic flow management (ATFM) of airports, considering the airport delay based on multivariate statistics. The uncertainty of effective measures in ATFM was estimated with multivariate probabilistic collocation method (PCM) in. The issue of determining the stochastic capacity of airspace in ATFM was discussed in. The heating effect of jet blast from 4 embarked aircrafts on take-off zone of the flight deck was studied in based on CFD technique and, similarly, the impact of the jet blast on the jet blast deflector (JBD) was discussed based on CFD theory in. Nevertheless, researches on the system modeling problems of ATFM model, like multiple feedback factors, time delay and complex time-varying, are not sufficient. In addition, the study on the differences of ATFM between the aircraft carrier and civil airport brought by different environments and platforms is also inadequate.
For example, due to the high risk of night recovery of embarked aircrafts, an accurate recovery schedule is necessary to ensure the security. However, if bolter or wave-off happens due to landing misses, the time schedule of recovery will be disrupted, recovery will be delayed, reduction of efficiency will be consequent, and the recovery security will be even harmed. In order to solve the existing problems in the previous models, a system dynamic method is applied in this study to build an ATFM model of embarked aircraft night recovery.
It is expected that it provides a viable solution to solve such ATFM problems. PREDICTION MODEL System dynamics is an integrated science of systems theory and computer simulation focusing on the system feedbacks and actions ( ).
It is introduced into various fields, such as economic, military, and ecological to solve the problems of complex non-linear giant systems with feedback structures (; ). The prediction model based on system dynamics theory has 4 parts: the analysis of night recovery process of embarked aircraft formation, the system boundaries determination and basic assumptions, stock flow diagram analysis and equation setups.
Figure 2 Vertical view of night recovery patterns for embarked aircrafts. Wave-off means the maneuver of nose-up for climbing of the embarked aircraft before touching the deck. It happens generally in adverse meteorological conditions of landing, inappropriate manipulating of the pilot, excessive deck motion etc. Bolter happens if the tail hook of the aircraft bouncing above the arrestor cable when touching the deck or the aircraft misses all the cables on the arresting area when landing.
In this case, the aircraft must be powered up immediately to climb for safety. THE DETERMINATION OF SYSTEM BOUNDARIES AND BASIC ASSUMPTIONS The system boundaries of the prediction model depend primarily on the scope and span of the variables and time, being determined according to the state variables. The subject of this paper is the number of embarked aircrafts at each phase of the night recovery.
Only the related entities are concerned, including the number of embarked aircrafts at the mentioned phases ( A - arrival; B - approach; C - final approach; D - deck landing; E - bolter or wave-off; ), deck arrestor gear area, flight deck landing area and temporary spots. The connections of the entities form the entire system discussed here.
Various uncertainties exist in the night recovery process during over-sea operations. The primary concerns of this research include key factors such as spinning of the embarked aircrafts, target acquisition with landing director radar and optical landing aid system, wave-off, bolter, touching the deck, arresting, and taxiing. The following assumptions are presented to simplify the prediction system. STOCK FLOW DIAGRAM ANALYSIS The stock flow diagram is built to distinguish the variables, clarify the logical relationships among the various elements, feedback forms and control laws of the system. It is a representation method with intuitive symbols for further research on the system.
Download free pointers on c reek pdf to excel. The stock flow diagram of the prediction system is a structural description of the arrival holding pattern, approach and final approach, landing route, wave-off, bolter, arresting gear area, deck landing area and temporary spots. It holds much more information than written statements can do and is clearer and more accurate in logic. The diagram consists of 9 state variables, 11 rate variables and 23 auxiliary variables. SIMULATION CASES AND ANALYSIS The model according to - were simulated in Vensim(r) Personal Learning Edition 5.9. In this section, the model of night recovery is simulated based on the Russian aircraft carrier Kuznetsov. The initial values of the factors of the prediction system are: L jc( t 0) = 0, L jj( t 0) = 0, L zhjj( t 0) = 0, L zjhx( t 0) = 0, L ff( t 0) = 0, L zlq( t 0) = 0, L lt( t 0) = 0, L lstj( t 0) = 0, m hs = 8, t jc = 10 min, n db = 8, l jc = 60 km, v jc = 800 km/h, l jj = 25 km, v jj = 380 km/h, l zhjj = 9 km, v zhjj = 280 km/h, t ffqr = 6 min, t tyqr = 6 min, λ ff = 0.2, t xhff = 10 s, l zjhx = 1 km, v zjhx = 240 km/h, λ ty = 0.3, t ty = 3 s, t zl = 3 s and t hx = 30 s. The control parameter t = 0 90 min.
Vensim
Figure 5 The quantity of embarked aircrafts at each stage without wave-off or bolter. (a) Embarked aircrafts before touching the flight deck; (b) Embarked aircrafts after touching the flight deck. Shows the quantity of embarked aircrafts during night recovery at the arrival, approach, final approach and landing pattern phases. At the arrival phase, embarked aircrafts are assumed to enter when t = 10 min and it is completed when t = 11 min; when t = 14.5 min, embarked aircrafts in the 8-aircraft formation begin to withdraw from spin holding pattern in turn, and the phase is finished when t = 22.5 min. The arrival phase has a range of 60 km for the spin holding pattern, and the withdrawing rate of aircrafts can be controlled at 1 aircraft per min.
When t = 14.5 min, the formation of embarked aircrafts enters the approach phase in turn. For the relatively small range about 25 km for the approach stage, a maximum of 4 aircrafts can be held in this phase. When t = 18.4 min, aircrafts will be captured by landing director radar and then enter the final approach phase. The whole approach phase will be finished at t = 26.4 min. The status at this phase becomes complex - the maximum number of aircrafts can reach 2, but for the aircrafts re-entering from wave-off or bolter, the quantity can be divided into 7 levels. The number reduces below 0.1 from t = 59.4 min.
The quantity of embarked aircrafts at the landing pattern phase is also complex - the phase begins at t = 20.4 min and, for the distance of landing pattern, it is about 1 km; the aircrafts only spend 0.25 min at a speed of 240 km/h in the pattern. Then the maximum number of aircrafts is 0.25 per min. The quantity of aircrafts of this phase also has 7 levels for the same reason of wave-off and bolter. The number of aircrafts reduces below 0.01 per min from t = 61.1 min. Shows the quantity of embarked aircrafts at each phase of the night recovery operations.
The case of wave-off and bolter makes the status complex and divides the quantity of embarked aircrafts into 7 levels. Embarked aircrafts enter the temporary spots when t = 21 min; when t = 45.1 min, the quantity of embarked aircrafts entering into the deck temporary spots is 7 and, when t = 64 min, that number reaches 7.8, which means that 97.5% of embarked aircrafts are recovered in probability. WITHOUT WAVE-OFF AND BOLTER The simulation results in the case without wave-off and bolter, which means λ ff = 0 and λ ty = 0 are shown in. It can be derived that, for an 8-aircraft formation, 12.5 min are spent at the arrival phase, 12 min at the approach phase, and the time spent at the final approach, landing pattern, arrestor gear area, landing area and temporary spots are 9.875; 8; 8; 8 and 10 min, respectively. The simulation results in both cases have a high reliability according to the capacity of ATFM of the Kuznetsov carrier during night recovery of embarked aircraft formation. CONCLUSIONS The prediction model of night recovery of embarked aircraft formation can provide receivable simulation results for air traffic forecasting in the case of bolter and wave-off to ensure that the flow of aircrafts at each phase of recovery is in accordance with the capacity of ATFM. The implement of air traffic schedule and flight plan can benefit from the simulation results so that delays, holding time and other bottlenecks of the recovery process will be minimized.
The results can provide the theoretical and technical basis for the development and implementation of ATFM of embarked aircrafts recovery. The following results were achieved through the research. REFERENCES Bertsimas D, Guglielmo L, Odoni A (2008) The air traffic flow management problem: an integer optimization approach. Proceedings of 13th International Conference; Bertinoro, Italy. Clarke JPB, Solak S, Ren L, Vela AE (2013) Determining stochastic airspace capacity for air traffic flow management.
Transport Sci 47(4):542-559. Doi: 10.1287/trsc.1120.0440. Dastidar RG, Frazzoli E (2011) A queueing network based approach to distributed aircraft carrier deck scheduling.
AIAA 2011-1514. Proceedings of the Infotech@Aerospace; St. DeArmo J, Wanke C, Greenbaum D (2010) Probabilistic ATFM: preliminary benefits analysis of an incremental solution approach; accessed 2017 Jan. Liu FQ, Hu MH (2011) Auctioning method for airspace congesting resource allocation and game equilibrium analysis. Transactions of Nanjing University of Aeronautics and Astronautics 31(3):282-293. Meng LH, Xu XH, Li SM (2012) Dynamic reroute planning under uncertain severe convective weather.
Journal of Southwest Jiaotong University 47(4):686-691. Mukherjee A, Hansen M (2009) A dynamic rerouting model for air traffic flow management. Transportation Research Part B: Methodological 43(1):159-171. Doi: 10.1016/j.trb.2008.05.011 Ryan JC, Cummings ML, Roy N, Banerjee A (2011) Designing an interactive local and global decision support system for aircraft carrier deck scheduling. AIAA 2011-1516.
Proceedings of the Infotech@Aerospace; St. Thompson KM, Tebbens RJD (2007) Eradication versus control for poliomyelitis: an economic analysis. Lancet 369(9570):1363-1371. Doi: 10.1016/S0140-672-7. Weigang L, Souza BB, Crespo AMF (2008) Decision support system in tactical air traffic flow management for air traffic flow controllers. J Air Transport Manag 14(6):329-336. Doi: 10.1016/j.jairtraman.2008.08.007.
Wesonga R (2015) Airport utility stochastic optimization models for air traffic flow management. Eur J Oper Res 242(3):999-1007. Doi: 10.1016/j.ejor.2014.10.042. Wolstenholme EF (2003) Towards the definition and use of a core set of archetypal structures in system dynamics. Syst Dynam Rev 19(1):7-26. Doi: 10.1002/sdr.259. Yang SW, Hu MH (2010) Robust optimization of aircraft arrival and departure flow allocation based on dynamic capacity.
Journal of Southwest Jiaotong University 45(2):261-267. Doi: 10.3969/j.issn.0258-2724.2010.02.017. Ye BJ, Hu MH (2010) Multi-airport ground holding problem based on airline schedule optimization: models and algorithm. Journal of Southwest Jiaotong University 45(3):464-469. Yue KZ, Sun YC, Liu H (2015a) Analysis of the flow field of carrier-based aircrafts exhaust jets impacting to the flight deck.
International Journal of Aeronautical and Space Sciences 16(1):1-7. Doi: 10.5139/IJASS.2015.16.1.1.
Yue KZ, Han W, Song Y (2011) System dynamics model on rapid supply of spare parts in carrier formation. Ordnance Industry Automation 30(7):27-32. Yue KZ, Cheng LL, Liu H (2015b) Analysis of jet blast impact of embarked aircraft on deck takeoff zone.
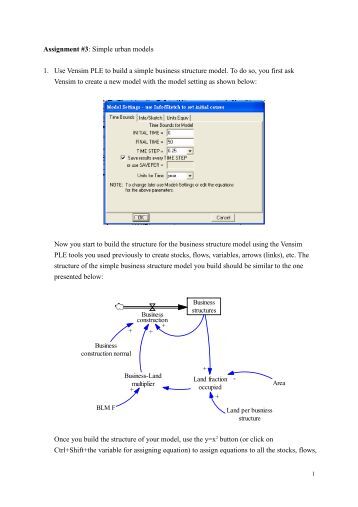
Aero Sci Tech 45(1):60-66. Doi: 10.1016/j.ast.2015.04.010. Yue KZ, Sun C, Luo MQ (2013a) Operation model on dynamic allocation system of embarked aircrafts. Journal of Beijing University of Aeronautics and Astronautics 39(8):1062-1068. Yue KZ, Sun C, Luo MQ (2013b) Operation model on the carrier warship recovering carrier aircraft.
Vensim Ple Download
Systems Engineering and Electronics 35(12):2527-2532. Zhang HH, Hu MH (2010) Collaborative allocation of capacity and slot in CDM ADGDP airport. Systems Engineering - Theory and Practice 30(10):1901-1908.
Zhang J, Hu MH, Zhang C (2009) Complexity research in air traffic management. Acta Aeronautica et Astronautica Sinica 30(11):2132-2142. Zhou Y, Wan Y, Roy S (2014) Multivariate probabilistic collocation method for effective uncertainty evaluation with application to air traffic flow management. IEEE Trans Syst Man Cybern Syst Hum 44(10):1347-1363. Doi: 10.1109/TSMC.20. Author for correspondence: Kuizhi Yue Naval Aeronautical University - Department of Airborne Vehicle Engineering - Staff Room of Aircraft 188 Erma Road Yantai/Shandong 264001 - China Email: AUTHOR'S CONTRIBUTION Conceptualization, Yue K and Cheng L; Methodology, Yue K, Cheng L, and Huang Z; Investigation, Cheng L and Huang Z; Writing - Original Draft, Yue K and Huang Z; Writing - Review & Editing, Cheng L and Yue K; Funding Acquisition, Yue K; Resources, Cheng L and Huang Z; Supervision, Yue K.